Case Study: A personal loan lender drives net loan growth while reducing risk
A Hong Kong licensed money lender partnered with CREDI AI to deploy its first AI-based credit scoring model. Learn how they were able to drive loan growth while reducing risks and losses.
What they faced before :
When they saw delinquencies for personal loans nearly double over a 3-year span, they already knew their scorecards did not work for their entire target customers. They made an adjustment by tightening approval policies and denied more customers by setting a higher score cut-off.
As they had struggles in net loan growth by their defensive policies, they were looking for a way to drive loan growth with confidence while reducing risks and losses.
While their executives understood the power of AI-driven credit decisioning, they were still using a traditional scorecard which was built more than 3 years ago and used only about 20 variables. They needed a help building and deploying its first AI-powered risk assessing model.
Mitigating risks with AI-powered credit scoring
They therefore decided to partner with CREDI AI to build a machine learning (ML) model which enabled them to extend credit access to more customers, while mitigating losses and the P&L impact.
CREDI AI and they created this ML-powered model using CREDI AI’s technologies and software. With the model, 477 predictive variables were identified, leveraging bureau data and application data which made them possible to better segment and assess risks clearly, eventually leading to 31% risk reduction. This reduction drives an estimated amount of HKD 32 million in annual savings.
With a stronger prediction power, they also enhanced pricing and increased loan amount based on growth targets. Their average loan size rose by 1.3x so far and they have reboosted portfolios growth safely.
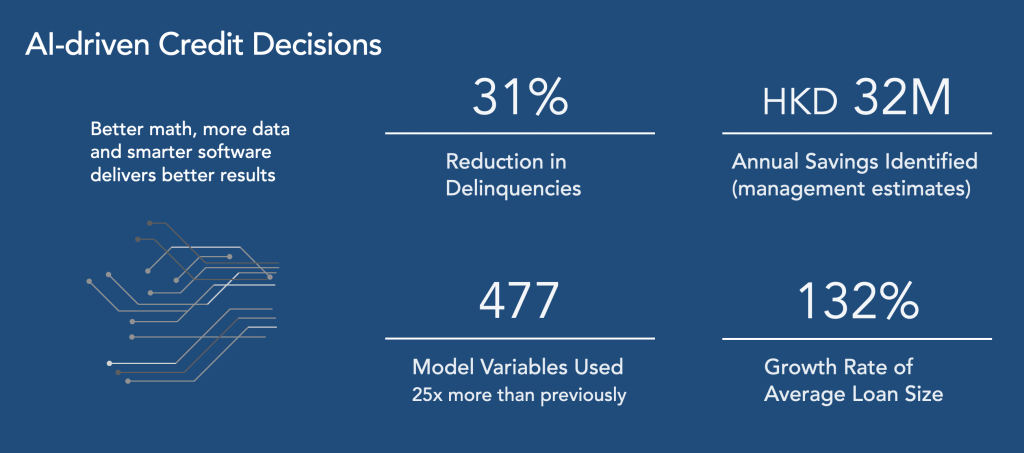
Using ML-powered credit scoring enabled them to reduce default risks, identify good borrowers and improve credit access for consumers, especially in near-prime or subprime segments who are main target customers for them. A clearer picture of borrower risks made loan decisions quicker and smarter.