2021 is the year for Hong Kong money lenders to look beyond traditional credit scores
In the US alone, 60 million adults are credit-invisible, this means, people has a limited or no credit history. Traditional credit scores are not available for these credit-invisible people, so they can’t help them to navigate financial inclusion because traditional scores can’t prove their creditworthiness.
In Hong Kong, the city is the international financial hub and matured consumer credit market however, there are still some people who have difficulties in accessing to the financial services they need. Millennials and Gen Z, workers from overseas and domestic helpers, many of them are either credit-invisible or thin-file and unable to measure their financial ability based on traditional credit scores.
Now, under the virus-driven recession, money lenders have tightened their loan decisions even for full-file customers though they are credit-visible. By relying too much on traditional data, their risk models are outdated and less predictable so they can’t measure customer’s risk precisely.
We’ve seen large/mid-size money lenders in Hong Kong who are still depending too much on traditional credit data. It’s about time to make alternative data available and unleash consumer’s creditworthiness. The impacts of COVID in consumer credit market will continue in 2021 so lenders need to re-model their credit scores by using alternative data under the current market changes.
In this article, we’d like to talk about how money lenders enable alternative data available for more customer acquisitions and better loan decisions.
Key takeaways in this blog:
- What is alternative data for better credit decisions?
- Requirements for alternative credit data
- How to obtain alternative data
- How alternative data enables better credit decisions?
What is alternative credit data?
Alternative credit data is information obtained from non-traditional data sources that helps to discover a consumer’s creditworthiness. While traditional data from credit bureau refers to an individual’s credit history, alternative credit data for credit scoring contains broader information that indicates a consumer’s financial ability and willingness to repay. Mobile payments, utility payments, rent payments, MPF, insurance payments can be alternative credit data since these data points help lenders to predict consumer’s financial capability. Employment history and salary record can be alternative data also. These employment data can indicate a financial stability when it comes to a credit scoring modelling.
Requirements for Alternative Credit Data
Alternative credit data for credit scoring comes from various sources; digital footprints, full-file public records and banking accounts data are some examples. There are some requirements for alternative credit data for lenders to make better predictions available.
According to research by Oliver Wyman, a good alternative data source should have the following characteristics.
- Coverage:
An alternative data source should have broad and consistent coverage.
- Specificity:
The source should provide information specifically about the individual.
- Accuracy and Timeliness:
Alternative data must be accurate and current or frequently updated.
- Predictive Power:
The information from the source should be relevant to the behavior being predicted.
- Orthogonality:
Ideally, alternative credit data should complement traditional credit bureau data.
- Regulatory Compliance:
Alternative data sources must comply with existing financial regulations.
How to Obtain Alternative Data for Better Lending?
Most of alternative data can be obtained in a traditional manner, by asking consumers. Loan applicants can be required to provide additional information by themselves. Money lenders can ask applicants to grant them access to account information from banks, credit cards, investments and mobile phones. Employment and salary data are also readily obtainable from applicants if lenders require them clearly as an application requirement.
Building partnership with various data aggregators is another way of obtaining alternative credit data. Partnering with a vendor or using a data aggregation platform enables money lenders to access to the alternative information about their users. Using aggregation technology can make the loan decision process faster. For building a better user experience by more efficient process, traditional lenders may choose to tie up with Fintech providers.
How Alternative Data Enables Better Credit Decisions?
Once money lenders are ready for collecting alternative data, the data then needs to plug-in credit scoring modelling. Machine learning can make use of numerous available data and track non-linear behavioural patterns even in unstructured data. ML algorithms can paint borrowers risk more clearly to identify patterns of applicant behaviour and ability for the repayment.
The more data and the better math lenders have, the more the borrower’s risk can be identified. Modern lenders can accelerate loan decision process by adopting more auto-decision when the model proves a high predictive power, so lenders can significantly reduce processing time and gain more customers regardless economic conditions.
According to FICO’s research, alternative credit data paints less value than traditional data, and traditional data alone, present more insights into a borrower’s creditworthiness. However, using alternative data in credit risk modeling is the only way for lenders to identify the credit-invisible customer’s ability to qualify a loan.
At CREDI AI, we also recommend money lenders to combine traditional data and alternative data into model development when it comes to risk model building. A prediction model built by using both traditional credit data and alternative data sources demonstrates a more predictive power. The combination of the two data sets enables loan decisions to be more accurate and more financial inclusion.
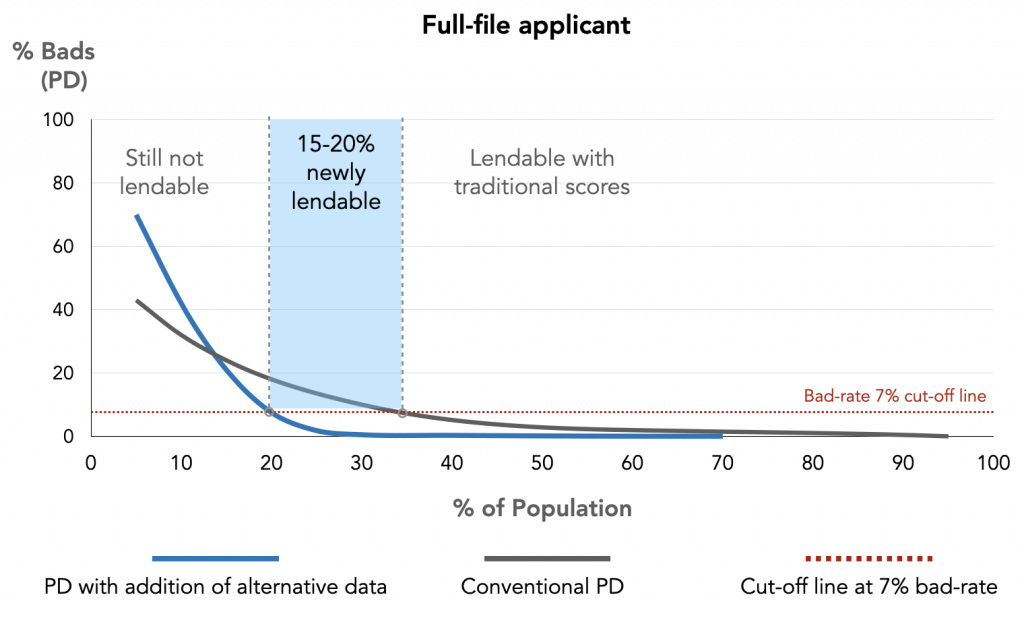
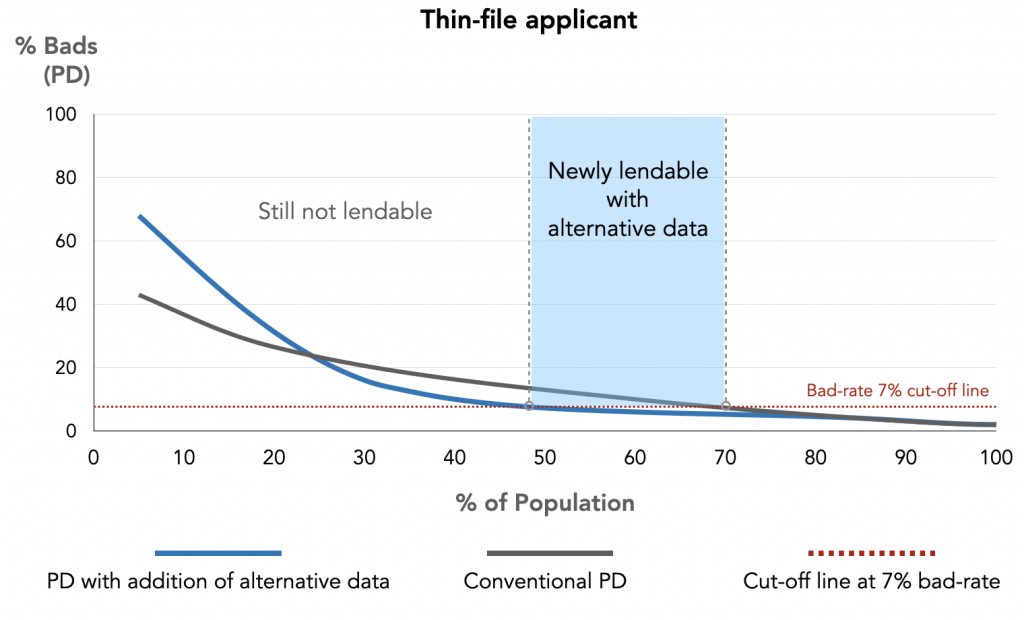
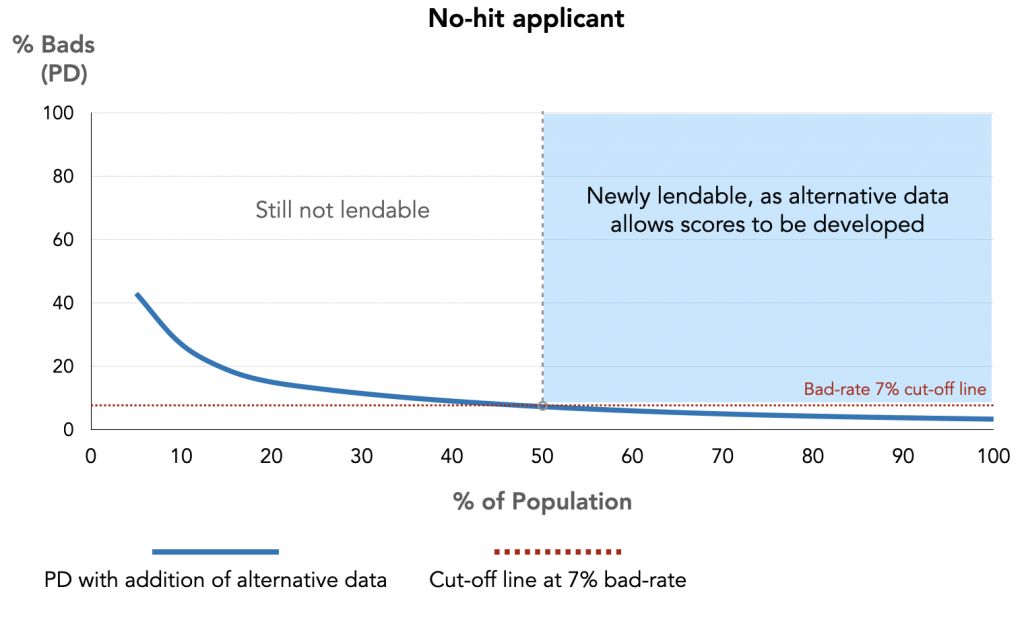
Source: Oliver Wyman Report
As the above three charts illustrate, a more accurate credit modelling by adding alternative data can significantly expand lendable populations. Adding more data and using better math enable lenders to paint more clear picture that can effectively separate goods and bads. Far more people now become lendable, the charts show the lendable populations in the blue area by adding of alternative data when the cut-off line is set at 7%.
With alternative credit data, money lenders can increase the number of their customers by improving their loan decisions. Moreover, alternative data enables lenders to build an accurate credit scoring for all types of applicants even for those credit-invisible by traditional data.
Regardless the economic conditions even in the downturn, money lenders can add alternative data and use machine learning modelling to acquire more customers with better loan decisions. In the difficult times with COVID, the situations have created an opportunity for Hong Kong money lenders to accelerate loan decision processes and win the future of lending.
A new world of consumer credit modelling has already begun. It’s time to make alternative data and new credit modelling available. At CREDI AI, we build high-performing prediction models for lenders. If you are interested to learn more about our service, contact us!