3 things money lenders need to know about data for machine learning models
Hong Kong’s jobless rate rose to 7.0% in January to the highest level near 17 years. Although the government has eased social distancing measures from middle February, the damages of job market and local businesses will be likely to remain as it will take more time for business activities to recover.
Global pandemic’s impact on the Hong Kong economy and lending industry has reinforced the urgent needs of digital transformation and move to AI. In the current environment, money lenders are not able to rely on credit scores that they have been using and they need a new way to assess credit risk more accurately.
AI becomes widespread for lending business, one of most impactful AI use cases is in credit risk underwriting. As money lenders are looking for more effective and faster ways in loan decisions, machine learning models will be the fundamental to adopt to the new normal.
As we have talked about how to make alternative credit data available for the money lenders for more customer acquisitions and better loan decisions in my previous post, the utilisation of data and AI will allow lenders of all sizes to model by using more variables and creating a clearer picture of applicant risk.
Money lenders using our machine learning technologies deploy up to 10 to 50 times more variables than they previously built models with traditional linear regression, or some of them did not use credit scores at all so they only relied on human decisions instead. That significantly changes their loan decision processes and improves in underwriting performance to make more good loans and fewer bad ones regardless economic conditions, even during a pandemic.
We also understand that switching to machine learning models raises tough challenges and lots of data questions: what kind, how much, data privacy, data preparation, etc. In this article, we’d like to share most common questions about data we get asked. We think these are essential things for money lenders to understand about the data when it comes to build machine learning models.
Key takeaways in this blog:
- What kind of data required for machine learning models?
- Should you use mobile data? How about social media data?
- How to manage data privacy in order to build machine learning models?
1. What kind of data required for machine learning models?
Typically, machine learning models rely on the following data sources. To be clear, no personal data is required in the datasets. All of identifiable information should be anonymised, removed or replaced with a mask character in practice. (More details follow in the below 3)
- Application data (must):
Based on application form by money lenders, the application data such as basic customer information is gathered from potential borrowers. Digital footprints with user behaviours will be utilized more commonly as online application has already become a standard.
- Historical loan performance data (must):
At CREDI AI, we normally require at least 1,000 historical loan performance data with a status, whether good or bad (delinquency – often 30/60/90 days past due). Lenders are not required to pre-process data at all.
- Bureau data (optional):
Traditional credit data provided by credit bureau. Some money lenders are non-Transunion lenders, so they don’t have such a bureau data at all. But not to worry, at CREDI AI, we can leverage machine learning as long as lenders have enough historical data as above-mentioned.
- Alternative credit data (optional):
In the case money lenders have already collected additional data from users such as mobile payments, utility payments, rent, MPF etc. These data points can help lenders to predict consumer’s financial capability and enhance credit scoring algorithm. If not yet, it would be good to start requesting for these alternative data.
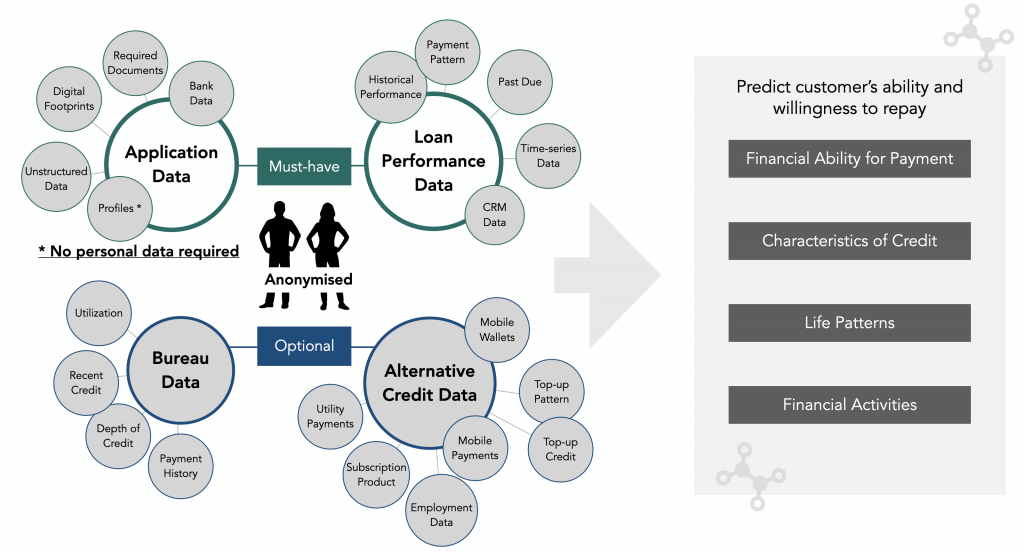
How to handle missing data?
In general, there are always missing data in the data source. Some data are only available if the customer applied online, or data went missing due to an upgrade or change in the data structure. But not to worry, machine learning models are really good at finding a way to relate even missing data in a meaningful way. An ensembled machine learning algorithm is able to handle the datasets which contain these missing data.
2. Should you use mobile data?
Indeed, our mobiles now contain a huge part of our lives. In today’s world of total connectivity, over-reliance on a single data source generates more limitations than opportunities. Money lenders could take advantage of mobile data to deploy credit scores especially for people with credit-invisible by traditional credit data.
However, most money lenders are not so accessible to mobile data unless they get involved in API ecosystem with gaining access to data sources by business partners. While almost all customers are already digital onboarding in several financial apps in Hong Kong, money lenders can simply request customers to self-report additional data that they have in their mobile.
- Historical data on mobile payments/top-ups
- P2P transfers/top-ups
- Information on loyalty points and cards
- Subscription services and recurring payment records
- Employment information and recurring payroll records
These data sources include credit variables to indicate customer’s financial ability and stability as above-mentioned alternative credit data. Customers are willing to share these information if money lenders provide questionnaires or forms to submit their data digitally and seamlessly by using their mobile.
How about social media data?
Not really. There are a lot of hype that social media such as Facebook contains insights of payment behaviours, but the truth is that social data does not provide a predictive signal which typical credit variables do.Utility payments data and bank data such as checking/saving accounts transactions embrace a lot of rich data that can build an accurate credit profile of a customer. Machine learning models don’t have to figure out if customers went shopping to buy luxury bags or where and when they went on their last vacation.
3. How to handle data privacy in order to build machine learning models?
Data privacy has been one of major topics that is discussed at every online meetings with lenders. But, as for data privacy and data security, those two terms are often mixed up when it comes to discuss about data protection.
Just to clarify, data privacy is not same as data security. To properly protect data and comply with data protection laws, you need to both data privacy and data security. Data privacy is about proper usage, collection, retention and storage of data. Data security is policies, methods, and means to secure personal data. (Read more about the difference between data privacy and data security.)
Data privacy is centered around how data should be collected, stored, managed and shared with any third parties as well as compliance with applicable privacy laws such as The Personal Data (Privacy) Ordinance or General Data Protection Regulation GDPR.
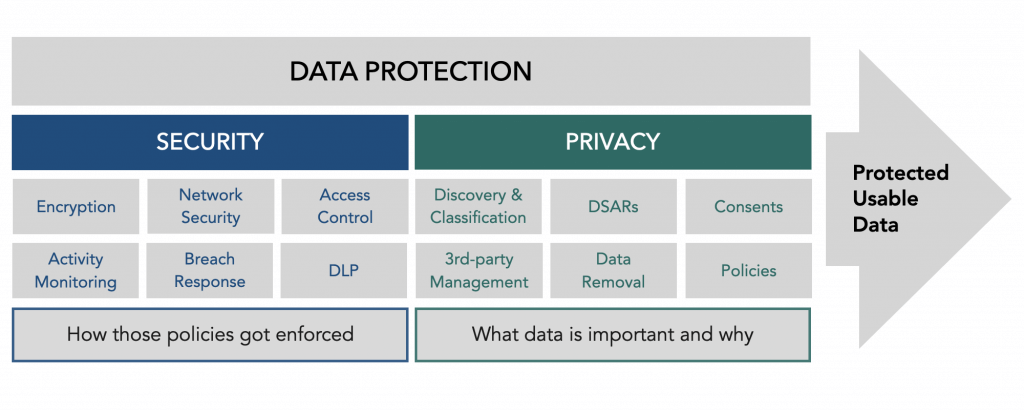
Source: Data Privacy Manager
Money lenders need to make sure how data should be shared with third parties like us when data is required to provide for building machine learning models based on the privacy laws. This is exactly the same question as the title, how to handle data privacy.
We don’t require any personal data which is personally identifiable information. Data anonymization is also applied if needed. There are some techniques of data anonymization such as character masking, data swapping, data substitution, generalization etc. No touchy subject of data privacy exists when we work with money lenders for sharing the data. This is quite a simple and normal thing, but just to mention as an essential part of handling matter properly.
Also about data security, data security is a prerequisite for data companies like us. We have set data security methods, practices and processes as strong as other data companies do such as encryption, multi-factor authentication, access control, network security, activity monitoring etc.
Hong Kong money lenders would face tough challenges in 2021 as emerging economic downturn. However, with the right understanding and appropriate approach to AI, money lenders can successfully implement machine learning models to demonstrate better performances even during a pandemic.
We’ve helped money lenders deploy machine learning models and efficiently deliver to better results. We like to share our expertise and best practices to navigate your move to AI. If you are interested to learn more about our service, contact us!