5 reasons why AI credit scoring has come under the money lenders’ spotlight
AI is already widely deployed in financial services and began as one of the most significant digital transformation drivers. Customer service automation, fraud detection and identity verification are the most commonly used applications of AI in finance.
Now, AI and machine learning (ML) have reached the central role of the lending industry: credit scoring for consumer lending. By detecting countless patterns in credit data, ML models enable lenders to paint a clearer picture of credit risk.
Using ML reduces default risks, identifies good borrowers and improves credit access for consumers, especially in near-prime or subprime segments which are main target customers for money lenders in Hong Kong.
This blog identifies near term AI-related trends to watch in 2021 and beyond. A transition to AI and ML underwriting is ongoing but faces some challenges for money lenders.
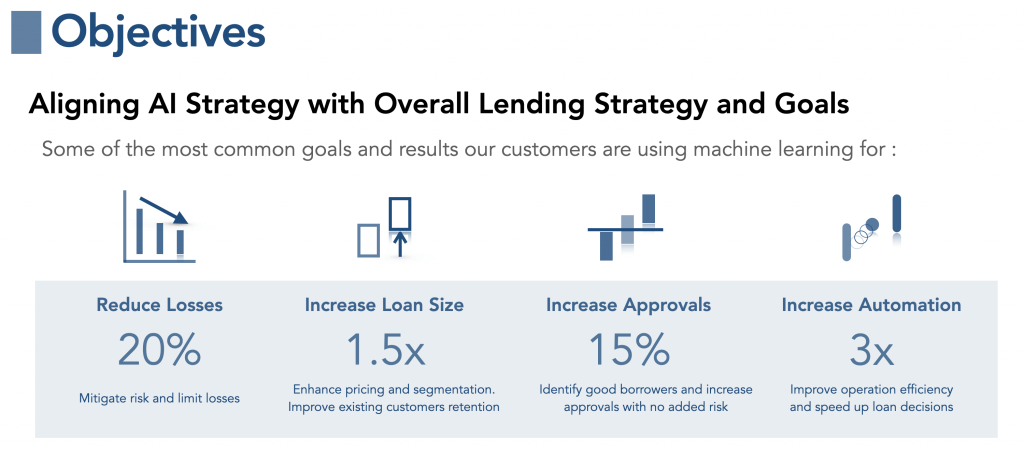
1. Agility becomes the highest priority due to the law reform
Through a long-standing debate on the old regime in the Money Lenders Ordinance (MLO), a cap rate will be lowering in the coming months. Money lenders are required to adapt that change very soon, most of them will have to take action speedily.
To identify good borrowers, reduce bad loans, cut operating expenses and strengthen lender yields are constantly business objectives, however agility now becomes more crucial to affect sustainability of the business. Although the economic has restarted to show a positive sign, money lenders are facing urgent tasks due to the reform of the law.
While money lenders may not have big data ecosystems and serious IT resources, they do have loyal customers with borrowing histories. Those rich customer profiles and their payment behaviors provide abundant data and hidden patterns to feed ML models which enable lenders to make smarter and faster loan decisions.
Money lenders can use fintech companies like us who have the capability to build and deploy custom ML models with agility and safety. Traditional way of re-modelling is a heavy-lifting and at time like now, money lenders have to take action quickly. This is where CREDI AI can help. It turns a year or more work to weeks.
2. Acquisition cost increases and customer retention becomes vital
Virtual banks in Hong Kong have already started a full-scale operation in the consumer credit market. As money lenders have already noticed, the competition has become more intense that offer higher customer acquisition costs in return.
Customers refuse to wait and choose a speedy one. This tends to become more obvious due to evolving the digital transformation. To identify good applicants and make instant loan decisions based on accurate predictions become more crucial than ever for money lenders of all sizes.
Competition is not limited to new customer acquisition. Customer retention is a definite factor in your lending strategy. Customers have a lot of refinancing options and easily to switch to competitors in the competitive market.
To improve customer retention, you have to look at your entire customer experience. The customer experience involves everything customers think and feel when they encounter your service. In today’s new reality, ML models carry a vital role both for retaining existing borrowers and acquiring new customers.
While ML models enable to increase approvals with no added risk, they can also uncover ways to enhance policies and rules for better customer journeys. These can be used to segment customer database, enhance pricing structure and identify existing customers who meet the desired retention criteria, i.e., their loan performance is acceptable, they are approaching a timing for an up-sale and what campaigns your marketing team can run.
3. Make the most of data for smarter loan decisions
As the pandemic is accelerating the rise of digital economy, the vast majority of interactions with customers must take place virtually in the post-pandemic era. Data and AI are reshaping lending so that money lenders are now required how they should utilize data and build risk models for smarter predictions.
AI has the capacity to ingest massive quantities of data. While traditional linear-regression models normally use 30 – 40 variables, ML models deploy hundreds of them. The more data and better math you have, the more clearly the borrower’s risk can be identified.
With ML models, your underwriting can become data-driven which means that your credit decisions make more accurate and faster by feeding more data – like digital footprints from digital marketing campaign and online application form, supplement data from applicants such as bank statements, employment data and mobile bills.
In general, the risk assessment method of money lenders places great importance on employment status so that their traditional risk models tend to heavily rely on financial stabilities based on employment information includes stable incomes.
As the digital economy accelerates diversity employment status also, like gig-workers such as contract workers, freelancers and part-time workers. Their incomes may not be as stable as traditional full-time jobs, however they may have enough financial capabilities for repayments.
Money lenders will be required to assess their risk better as traditional models usually overlook their financial abilities. One of the most predictive data is cashflow data – such as records of transactions in and out of consumers’ bank accounts – because it provides a comprehensive picture of applicants’ economic behaviors.
ML models can handle unstructured data well and also extract the most critical data from larger data sets efficiently. But if you don’t have such larger data sets, not to worry. ML models can help lenders squeeze more insights from the existing data that you already have.
4. Financial inclusion will be enforced
As Hong Kong’s consumer credit market has heavily relied on a single credit bureau for long years, consequently the situation generated some serious problems and now the government has addressed to build the new operating model by multiple credit reference agencies.
The city has about 2,300 licensed money lenders, however more than 90% of them are not able to access to the credit bureau data. There are several conceivable reasons for this, but one of serious problems is many money lenders are left remained out of the bureau data.
The government-led new scheme might encourage credit data inclusion for all licensed lenders so that more lenders will be required to access and report to credit bureaus in the near future. The new scheme is scheduled to launch in Q4 2022.
For a healthier industry growth and fairer market competition, the credit data inclusion should be enforced. And simultaneously, this will be followed by generating opportunities of more financial inclusion especially for thin-file and no-hit applicants like Gen-Z and domestic helpers.
With AI and ML models, financial inclusion can be accelerated by deploying more variables both credit data and alternative data – like bank account information, mobile payments, utility payment history – to supplement applicant profiles.
5. Fair lending will be valued
In Hong Kong, the lending ordinance and regulation are still not so strict if compared to other countries. As above-mentioned in the 1 and 4, the government has already addressed to modernize those issues, however there is still room to improve the lending practices.
A fair lending, the term would be not so commonly used in the Hong Kong lending industry. In US, the government and regulators have strictly controlled fair lending laws and compliances as credit discrimination is one of social problems has yet to solve.
Although the backgrounds and situations completely differed by countries/regions, fair lending might be a common challenge for all nations. Credit discrimination is often hidden or even unintentional, which makes it hard to spot. For example, when your lending denied credit to your applicants without giving a reason. This can be a red flag. Consumers should have a right to know a reason for the denial.
ML models help lenders to eliminate unnecessarily biases from your loan decisions and provide insights to explain which variables impact the credit scores. Transparent is going to be essential for AI in credit decision. No matter how consumers and regulators are going to demand, using ML models in credit underwriting that should be responsible both to business goals and requirements with transparency.
CREDI AI is your AI credit engine to achieve your business goals and take your lending to the next levels.